Plenary Speakers
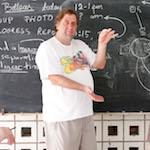
Bruce Reed
National Institute of Informatics, Tokyo, Japan
- Title: Random Models Of 21st Century Networks And Their Connectivity Structure
- Abstract: The traditional Erdos-Renyi model of a random network is of little use in modeling the type of complex networks which modern researchers study. It postulates that each node has the same likelihood of being attached to every other node. However, in, e.g. the web, certain authoritative pages will have many more links entering them. A 1995 paper of Molloy and Reed, cited over 1500 times, sets out some conditions guaranteeing the existence of a giant component in a graph with a specified degree sequence. This work has attracted such a great deal of attention because it can be applied to random models of a wide range of complicated 21st century networks such as the web or biological networks operating at a sub-molecular level. A heuristic argument suggests that a giant component will exist provided the sum of the squares of the degrees of the vertices of the network is at least twice the sum of the degrees. Molloy and Reed proved that this is indeed true subject to certain technical conditions. Many authors, have obtained related results by specifying different technical conditions, or by tying down the size of the giant component. Since the interest in this result is its wide applicability, it is natural to try and prove it under as few assumptions as possible. Recently, Joos, Perarnau-Llobet, Rautenbach, and Reed proved the result under essentially no conditions. I will present, in an accessible way, a variety of complex networks and their random models to which the Molloy Reed result has been applied. I will then sketch briefly the proof of our result and how it differs from the proof of the Molloy-Reed result.
- Bio: Professor Reed received his degrees in Mathematics and in Computer Science at McGill University. Following postdoctoral fellowships and faculty positions in Europe, Canada and the USA, he joined the faculty of McGill University in 2001. He held a Canada Research Chair in Graph Theory from 2001-2015, was elected as a Fellow of the Royal Society of Canada in 2009, and won the 2013 PIMS-CRM-FIELDS Prize. His 1995 paper with Molloy on random networks with a given degree sequence has been cited over 1500 times and reprinted in a volume setting out seminal contributions in the area.
- Web page: http://cgm.cs.mcgill.ca/~breed/
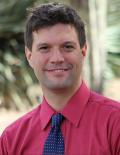
Adam Douglas Henry
School of Government and Public Policy, University of Arizona
- Title: Social Learning in a Segregated World
- Abstract: The connectedness of the modern world provides unprecedented opportunities for social learning --- that is, the adoption of new values, beliefs, and behaviors that enable human agents to become more informed and effective problem-solvers. Developing better theories of learning is an important endeavor in the social sciences and has applications across a wide range of human behaviors, such as the formation of opinions, the evolution of cooperation, and the use (and misuse) of science in the policy process. Unsurprisingly, networks arising from the World Wide Web play an important role in learning as these networks provide platforms for social interaction and information search. While a growing, increasingly connected Web Graph creates opportunities for learning, the structure of many real-world networks tends to limit exposure to diverse information and viewpoints. The ability of people to customize their networks based on their individual interests, tastes, and biases creates a fundamental problem for learning: Having diverse networks is thought to be a prerequisite for learning, however many networks are "segregated" in that network ties are strongly correlated with attribute similarity. So while we live in an increasingly diverse world, people are increasingly embedded in homogenous social environments. This presentation will explore the contributions of network models to the development of better theories of learning. Data on several different types of networks are used to examine three central propositions, including 1) real-world networks exhibit segregation, 2) segregation is an impediment to learning, and 3) segregation arises as a result of homophily, or the tendency for network actors to form ties with others who are similar. Models of network evolution can help us to discover strategies that will help actors to become more effective learners within complex, networked systems.
- Bio: Adam Douglas Henry is an Associate Professor in the School of Government and Public Policy at the University of Arizona, and is an affiliated member of the University of Arizona's Institute of the Environment. Prior to joining the University of Arizona faculty, Henry completed his PhD at the University of California, Davis, and was a research fellow at the Kennedy School of Government at Harvard University. He uses network analysis to study questions of environmental policy, particularly how actors in the policy process learn strategies to address complex and emerging problems of environmental sustainability. His work advances basic theories of how individuals form networks with one another, how learning occurs within networks, and how networks evolve over time. His work has been funded by the U.S. National Science Foundation, the U.S. Department of Energy, and the National Renewable Energy Laboratory. His research uses methods of agent-based simulation and mathematical modeling in additional to the statistical analysis of networks.
- Web page: https://sites.google.com/site/adamdouglashenry/